Can we build sandcastles with how apparently worthless data,
The Internet of things, big data and prescriptive maintenance are supposed to be the big promises from the 21st century. No more unplanned downtime because we can predict all failures. Many companies, however, struggle with these developments. They say there is hardly any data available and question the usability. It appears to be “loose sand” … until you start working with it.
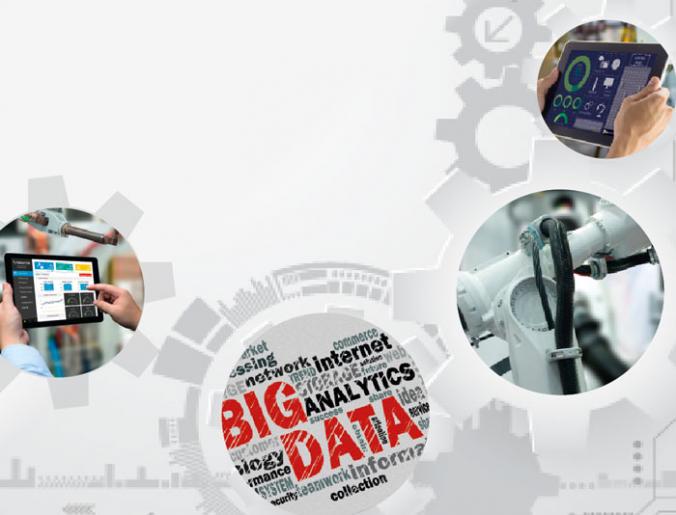
The field of maintenance is developing. More and more, maintenance and asset management are mentioned in the same breath. Where maintenance focuses mainly on the present and nearby future – the assets need to function to be able to deliver the required production or service – asset management is more about long-term planning, life-cycle issues, and moder-
nization. The goal is to monitor the lifespan of the assets and start investment projects at the right time to guarantee safety and reliability.
Poor data quality?
Executing asset management requires knowledge about the expected lifespan, use, degradation and, eventually, failure of assets.
– Our experience is that companies that think they only have low-quality data, at least have these figures at their disposal, says Peter Decaigny of Mainnovation.
– And they are more useful than you think.
Companies that are ruled by the illusion of the day – they are operating like a fire brigade with a focus on corrective maintenance – struggle to see the big picture.
– Everyone is busy, malfunctions regularly occur and most attention is paid to solving them as quickly as possible. The misconception is that registration of this downtime is just 'loose sand'. However, if you compare the downtime (in hours) with the frequency of failure - the number of failures - you gain insight into which assets often cause downtime for a long time.
These are the assets you need to pay attention to, Decaigny says.
– By looking for the cause or reason for the downtime, perhaps they can be resolved or removed.
– We gain insight into the 'Mean Time Between Failure' and also the 'Mean Time to Repair' and this helps to draw the right conclusions.
Incoherent?
In another case there is a fair amount of data available, but there seems to be no connection. It seems like an incoherent story from which no conclusions can be drawn. But here too the message is 'just start with what you do know'.
The first step is collecting and analysing data. If the analysis only raises questions and does not provide any insight, it is advisable to take a critical look at the representation of those figures.
– We can, for example, plot the Time to Failure in chronological order. So how many weeks did it take for an asset to fail. This could just result in an arbitrary number of figures. From 120 weeks, to 40 weeks, to 16 weeks and then suddenly 118 weeks again.
It is difficult to draw conclusions from this. We see companies making the mistake of taking an average. In this example, one would replace an asset before the 74th week, but is that 'just in time' or is this capital destruction? Data can often initially lead to confusion instead of insight.
– But don't give up, Decaigny says.
– Start to combine data. Use other models that compare different values to the Time to Failure. Or focus on the peaks. What causes the outliers?
Big data
Mainnovation has clients within plants, fleet and infra. This means great versatility in assets. From power stations, tank storage companies and industrial installations, to transport and various infrastructure companies.
– Every asset is unique. Even comparable assets have unique factors such as the method of use, the level of maintenance and the skills and tools of the operators and the technical service are of influence, explains Decaigny.
– Furthermore, there are, for example, weather influences or the pressure or humidity that can have negative effects on the materials. We know the term 'a Monday morning product', but that should be adapted to ‘a Tuesday afternoon product’, because statistically that turns out to be a bad production moment. How come? Nobody knows.
With this Decaigny wants to emphasize that we cannot just blindly rely on numbers.
– It starts with data. And by working with it you can improve the data. Then it will become clear that the factor 'temperature' – as an example – must also be taken into account. And who knows, you might discover that the Thursday afternoon operator prefers to work with an open window.
Value drivers
A correct analysis of the available data is therefore of great importance. Not only when minimal data is available, but also when we use big data and take various external factors into account. Moreover, it becomes increasingly difficult to compare apples to apples and to draw conclusions.
Another approach to get started with data is to first determine what the most important value driver is. In other words: what should we aim for in order to create value with maintenance and make a positive contribution to the operating result.
– That can differ per company, even per factory, explains Decaigny.
– While one wants to aim for maximum uptime, because the demand for the product is very high, the other may have to focus on cost reduction. There is also value in reducing (security) risks or perhaps it is better to invest in modernization now because this has economic added value in the long term.
These are the four value drivers from Mainnovation's VDMXL methodology.
– And whoever wants to steer in four directions, will eventually come to a standstill, so that's never a good idea.
Compare
In the first example we mentioned in this article – where it was all about minimizing downtime – the focus was on asset utilization and improving uptime. In this case we opt for the value driver cost control. If this is the mission, we should collect data to help make decisions about operational expenditure (OPEX). But how do you know whether these costs are too high and can possibly be reduced?
Decaigny has a simple answer to this question:
– Compare, for instance by benchmarking.
Mainnovation has a benchmark database of more than 1.000 companies. Comparing data with companies in the same industry, provides a realistic picture of the improvement potential. By dividing the investment amount by the replacement value, large companies and small companies can still be compared.
– For this big data is not a must. But it is important to choose the right data. By comparing apples to apples, you know where you stand and what the possibilities are to take steps forward to bring you closer to your business goal, states Decaigny.
So, we have seen that it is good to take the plunge and just get started. Even if it seems like loose sand, it is possible to build sandcastles.
A final tip that Decaigny also likes to give is:
– Make it visible. Let the shopfloor participate. Show the data, show the improvements so that people also understand which buttons they have to turn – literally or figuratively – to get even more positive figures.