Part III: AI Factory for Operation
All over the world, industries are boarding the digital train and exploiting the power of new and emerging technologies, such as Artificial Intelligence (AI), Deep Learning (DL), Machine Learning (ML), Big Data analytics, and Digital Twins, to enhance competitiveness and make operations competitive and economically viable.
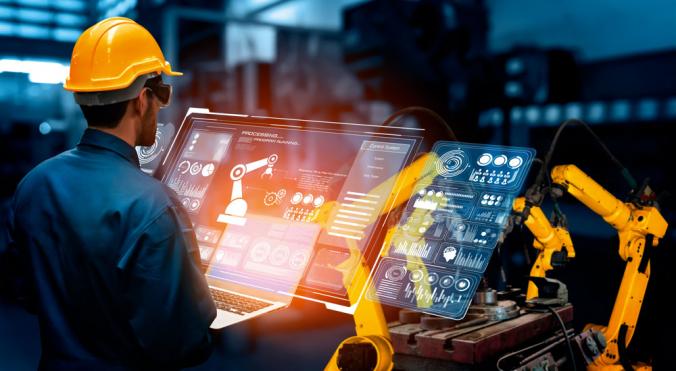
However, transitioning to digitalisation to manage the complex engineering assets effectively and efficiently is a challenging task, as it requires the use and integration of various tools, technologies and models.
The move to implementation of new and emerging technologies requires availability and accessibility to data and information, as well as domain-specific physics-based models to gain deeper insight into industrial processes, thus facilitating correct decision-making by considering all relevant and contextual information. In this transformation journey, data and models are considered digital assets (ISO55000) that reflect the current health of an asset; and processing such data permits a glimpse into the future trends of an asset’s health and its performance from a life cycle perspective.
However, to establish effective and efficient asset management using a life cycle perspective and to exploit the power of new technologies, we need appropriate concepts, methodologies, tools, and technologies, especially given the requirements of different types of assets and the associated domain-specific challenges. More specifically, industries are facing challenges developing and implementing AI to increase the level of autonomy in maintenance, especially in the contexts of maintenance and repair needs assessment, task planning, resource allocation, task execution, performance assessment, and continuous improvements, whilst considering the relevant contextual information.
The digital transformation of industry requires deeper insights into the operational domain of assets, prevalent business and governance models, and regulatory regimes to adequately address the challenges. From a digital asset management perspective, the main challenges are related to source integration, distributed computing, content processing, and cybersecurity.
A technology platform could bridge research findings with innovative solutions that are scalable for the transformation of industries. These platforms could provide digital pipelines between data providers and data consumers, as illustrated in Figure 1. We label this concept the AI Factory (AIF). Each pipeline represents a set of orchestrated activities aimed to extract, transfer, load, and process data between the provider and the consumer; pipelines are configurable entities, which can utilise a palette of technologies for communication, storage, and processing to enable context-adaptability and meet the users’ requirements. The selection of appropriate technologies for each pipeline should be based on the context-specific requirements such as requirements for scalability, authentication, and authorisation.
To facilitate the development of line-of-production and to select the appropriate technologies in the AI Factory platform, an Analytics Autonomy Level (AAL) needs to be developed; see Figure 2. The AAL aims to identify the maturity level of analytics in an organisation aiming for industrial AI. When the digital and AI maturity of an organisation are identified, a roadmap can be developed to support a data-driven approach based on the current needs and future opportunities.
AI Factory (AIF:) Content and products
The AI Factory is a virtual technology platform which integrates technologies and tools to facilitate engineering and business solutions by exploiting the power of data and data-driven technologies. Of course, organisations have been using data in their decision-making processes for centuries, but an AI factory platform also considers contextual information enhancing the quality of decision-making process. Furthermore, the AI Factory also integrates and uses models based on physic-of-failure to develop engineering and business solutions through a use-case approach.
Digitalisation and the emerging technologies have made an enormous difference to the way data is collected and used for decision-making, and this provides the foundation for the structure of AI Factory and its architecture.
First, digitalisation has enabled a set of digital infrastructures in society, industry, and transport that can be used in various contexts, such as sharing data and models. Second, computing technologies have given society, industry, and transport (among others) the ability to run on a digital infrastructure. Third, Internet of Things (IoT) and sensor technology have augmented human senses with the capability to sense and measure new phenomena, provided as data flowing throughout the digital infrastructures. Finally, AI has enabled us to discover knowledge from a vast amount of data, using a digital infrastructure and computing power. These four ingredients have changed the concept of the data-driven approach.
Today, the data-driven approach is associated with complex fact-based decision-making processes using digital infrastructures, distributed computing (cloud/edge), sensor data, and augmented analytics empowered by AI. The fundamental idea of the data-driven approach is the same: making decisions on facts (data).
In many areas, including the maintenance of transport systems, the data-driven approach promises better accuracy via fact-based decision-making, leading to operations excellence and system sustainability with respect to economy, technology, and the environment. However, the mere availability and accessibility of data provided by an appropriate information logistics system is not sufficient for good maintenance decision-making. Data needs to be processed, analysed, and interpreted using digitalisation and AI technologies.
Context awareness is another important aspect which needs to be considered in maintenance decision-making. Context-awareness is the ability to sense the context in which a decision will be made, and adapt the analytics and information logistics to support the decision-making process.
The following essential aspects must be considered in a data-driven approach:
- Information logistics, representing the digital infrastructure
- Analytics, representing knowledge discovery through a set of algorithms, fed by data from the information logistic infrastructure
- Context-awareness, representing a set of situations for which decisions will be made, i.e., the purpose of analytics.
To improve the accuracy of analytic services, the AI Factory implements an architecture that integrates model-driven and data-driven approaches in a seamless manner, as illustrated in Figure 3
AI Factory for Operation and Maintenance
The ongoing digitalisation and implementation of AI-technologies in operations and maintenance depend on the availability and accessibility of data for geographically distributed systems. Lulea University of Technology has created AI Factory (AIF), a seamless platform built on loosely coupled storage and computing services for data sharing. AIF is a set of smart cloud/edge-based data services that aim to accelerate digitalisation in industry and transport. AIF services provide capabilities such as acquisition, integration, transformation, and processing of asset-related data across all stakeholders, where services can be invoked on-premise or in multiple cloud-based environments. AIF is applicable to numerous industries. For example, AI Factory for Railway integrates technologies and business requirements and other historical and contextual information to gain deeper insight into the performance of railway assets.
AIFs overall architecture rests on four main pillars; see Figure 1:
- A technology platform
- A digital governance platform (eGovernance)
- A communication platform
- A coordinating platform
An important aspect to consider when developing decision-support solutions based on AI and digital technology is the users’ experience. The user experience (UX) design process aims to create relevance, context-awareness, and meaningfulness for end-users. In railway contexts, applying a human-centric model in the development of AI-based artefacts will enhance the usability of the solution, with a subsequent positive impact on decision-making processes. Therefore, the analytics services in AI Factory are combined with other technologies such as Virtual Reality (VR), Augmented Reality, Mixed Reality (MR), and so on, to improve user experience (UX) in AI implementation.
Ramin Karim, Diego Galar and Uday Kumar,
Division of Operation and Maintenance Engineering Luleå University of Technology