Measuring the Value of Data in Maintenance
It is easy for organizations to assume that more data equals more value in maintenance. However the value of data is case dependent and should be assessed to ensure that the benefits from the data exceed the additional costs.
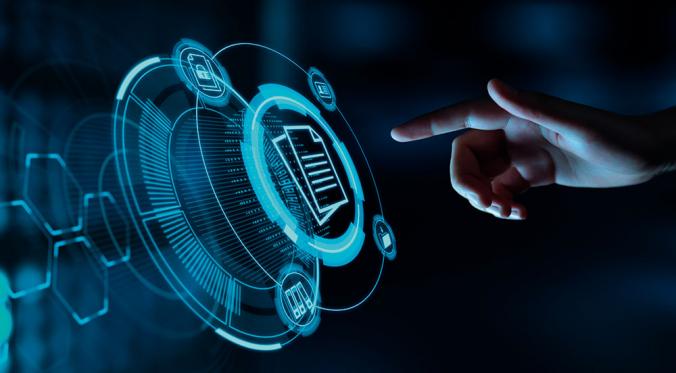
Many maintenance organizations have been tempted by the big data hype into collecting excessive amounts of data without specific business cases or data exploitation plans. When following the hype, it is easy to forget that the additional value created by the data must exceed the costs of resources used to collect and analyze it (Günther et al. 2017).
Achieving significant value from big data tends to require extensive resource use, however, many companies do not have the necessary resources and competence to keep experimenting with big data technologies, especially within the manufacturing and maintenance industries where the technology maturity is currently quite low (Diez-Olivan et al. 2019; Kans 2013). It has been acknowledged that the optimal amount of data in maintenance decision-making depends on the size, business, competences, and complexity of assets and processes within the organization (BS ISO 55001 2014).
The value of maintenance data also depends on the situation: for instance, in corrective maintenance, data are mostly used to detect failures and to decide whether to repair the asset immediately or at a later date, whereas in condition-based maintenance the data are considerably more complex and used to define measurement parameters, techniques and locations, maintenance action limits and maintenance actions.
Value of data
Value of data can be defined as having the right information, in the right amount, quality, format, time, place, and for an appropriate price (Bucherer & Uckelmann 2011). Familiar mostly as a production philosophy, lean management emphasizes increasing value through eliminating waste (Gupta et al. 2016).
Adapted to data management, lean could help maintenance organizations in assessing and maximizing the value of their data-based decision making (Marttonen-Arola & Baglee 2019a). The waste types in data management include:
- Unnecessary data (duplicate, non-relevant or too detailed data which can cause an information overload)
- Unnecessary transfer of data (non-value adding transfer between people, systems or organizations)
- Unnecessary processing of data (non-value adding processing, e.g. changing format, ensuring access, copying, unnecessary summarizing)
- Underutilization of data management resources (for example unused IT systems or personnel)
- Poor quality data and/or analyses (can lead to suboptimal decision making)
- Waiting for data or missing data (waiting or looking for data items) (Marttonen-Arola & Baglee 2019b)
To assess the value of maintenance data, quantifying the changes in the aforementioned wastes is beneficial. Figure 1 shows how the value of additional maintenance data can be modelled based on various types of decreasing waste in the data management process. A number of the waste types can be quantified in terms of time, which makes evaluating the overall value quicker. The quality of data and analyses can be taken into account by multiplying the decrease of waiting for data by an uncertainty factor. This reflects that the benefits of exploiting data in decision-making may be lost if the data are unreliable.
Applications in maintenance decision-making
To demonstrate applying the above-presented approach in maintenance decision-making, an industrial example from an automotive part manufacturing company is presented. The company has a manual data collection process and conducts mostly corrective maintenance on their production lines. The data which are currently collected are production-led and either not used to support maintenance management decisions or the data is used with little effect. For example, historic and useful data on the root causes of failures are not recorded. The maintenance manager of the company is considering expanding the data collection and implementing a Computerized Maintenance Management System (CMMS). They would first pilot the system on three of their 15 production lines.
The financial value of the investment (including the CMMS as well as additional data gathering and analyses) was assessed (see Figure 2). The time used in gathering, processing and analyzing the data would increase significantly if the company invested in more data. The costs of the CMMS software are taken into account as underutilized data management resources, because at first the software would only be used for the data of the three specific pilot production lines. Regarding the benefits of the investment, adopting the CMMS would decrease the amount of unnecessary data transfer. Currently the maintenance staff are required to insert the data manually into electronic spreadsheets every day. However, the investment appraisal concluded that with corrective maintenance, the company would not benefit from the additional data and the CMMS in terms of actual maintenance costs or the value of lost production. Thus the benefits of the investment would not be able to cover the additional costs.
The company has also expressed their interest towards increasing the role of predetermined maintenance in their production. Another investment appraisal was conducted to see if a change in the maintenance approach would affect the profitability of the investment. In this case the additional data collected to the CMMS could be exploited to design predetermined maintenance schedules and prevent asset breakdowns. Figure 3 shows the value of the investment if the data was successfully used to achieve moderate breakdown prevention rates at the three pilot production lines. The reliability of the data and the analyses has been assumed to improve slightly due to severe inaccuracies in the current manual system (including poor legibility of the forms, inconsistent terminology and clearly incorrect data). It is clear that with predetermined maintenance, the significant decrease of lost production would justify the investment.
This example showed that it is crucial for companies to assess the value of maintenance data before investing in additional data and IT systems. Data only have value when exploited, and the additional costs of gathering, storing and analyzing the data must be exceeded by the additional benefits in maintenance. Although the value of data is challenging to measure, even rough quantitative assessments would help the decision makers.
This article is part of LeaD4Value research project. More information: https://lead4value.wordpress.com/ .