Industrial AI in maintenance: false hopes or real achievements?
Artificial intelligence (AI) is an umbrella term for a set of technologies in which computer systems are programmed to exhibit complex behaviour in challenging environments. AI is regarded as the major force driving innovation today.
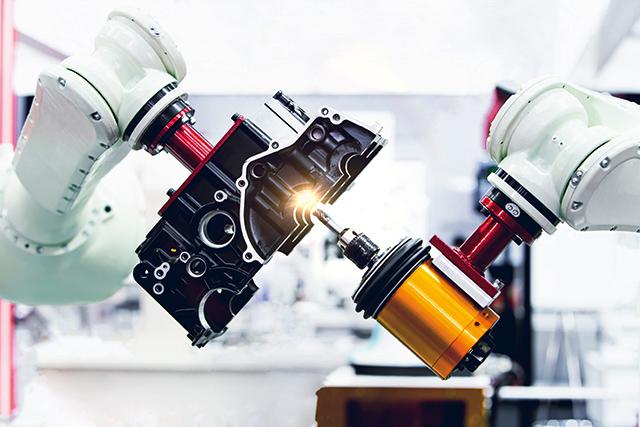
From an industrial point of view, AI technologies should be understood as methods and procedures that enable technical systems to perceive their environments through context and situation awareness. They are able to process what they have monitored and modelled, solve certain problems, find novel solutions never found by humans, make decisions, and learn from experience to be better able to manage the processes and tasks put under AI supervision, Figure 1.
Machine learning (ML) is one area of artificial intelligence used by industry. Machines need data to learn, either large quantities of data for one-time analytical purposes, or streams of data from which learning is continuously taking place. Based on acquired data either on line or off line, machine learning can reduce complexity and detect events or patterns, make predictions, or enable actions to be taken without explicit programming in the form of the usual ‘if-then’ routines or without classic automation and control engineering, Figure 2.
AI technologies are expected to increase the efficiency and effectiveness of industrial processes. The primary goals are to reduce costs, save time, improve quality, and enhance the robustness of industrial processes. However, AI is not as well-used in industry as we might expect, given its potential. Enormous changes and high costs are needed to integrate AI applications into corporate structures and along the entire value-added chain. At this point, AI applications tend to be found in the areas of robotics, knowledge management, quality control, and maintenance analytics shifting from traditional approaches to predictive ones.
A good field for AI in maintenance in industrial environments is the analysis and interpretation of sensor data, distributed throughout equipment and facilities. The Internet of Things (IoT), i.e. distributed data-suppliers and data-users capable of communicating with each other, is the basis for this use of AI. IoT acquires the data after pre-processing, records the status of all different aspects of the machines, and performs actions in process workflows on the basis of its analysis. Its central purpose is to identify correlations that are not obvious to humans to enable predictive maintenance (Figure 3), for example, when complex interrelated mechanical setting parameters have to be adjusted in response to fluctuating conditions in the environment to avoid compromising the asset’s health.
Industrial AI’s capacity to analyze very large amounts of high-dimensional data can change the current maintenance paradigm and shift from preventive maintenance systems to new levels. The key challenge, however, is operationalizing predictive maintenance, and this is much more than connecting assets to an AI platform, streaming data, and analyzing those data. By integrating conventional data such as vibration, current or temperature with unconventional additional data, such as audio and image data, including relatively cheap transducers such as microphones and cameras, Industrial AI can enhance or even replace more traditional methods. AI’s ability to predict failures and allow planned interventions can be used to reduce downtime and operating costs while improving production yield. For example, AI can extend the life of an asset beyond what is possible using traditional analytics techniques by combining data information from designer and manufacturer, maintenance history, and Internet of Things (IoT) sensor data from end users, such as anomaly detection in engine-vibration data, images and video of engine condition. This information fusion during the lifecycle of the asset is called product lifecycle management (PLM).
Explainable AI in Maintenance
Advances in AI for maintenance analytics are often tied to advances in statistical techniques. These tend to be extremely complex, leveraging vast amounts of data and complex algorithms to identify patterns and make predictions. This complexity, coupled with the statistical nature of the relationships between input data that the asset provides, makes them difficult to understand, even for expert users, including the system developers, Figure 4. This makes explainability a major concern.
While increasing the explainability of AI systems can be beneficial for many reasons, there are challenges in implementing explainable AI. Different users require different forms of explanation in different contexts, and different contexts give rise to different needs. To understand how an AI system works in the maintenance domain, users might wish to know which data the system is using, the provenance of those data, and why they were selected; how the model and prediction work, and which factors influence a maintenance decision; and why a particular output is obtained. To understand what type of explanation is necessary, careful stakeholder engagement and well-thought-out system design are both necessary as can be seen in figure 5.
There are various approaches to creating interpretable systems. Some AI is interpretable by design; these systems tend to be kept relatively simple. An issue with them is that they cannot get as much customization from vast amounts of data as more complex techniques, such as deep learning. This creates a performance-accuracy trade-off in some settings, and the systems might not be desirable for those applications where high accuracy is prized. In other words, maintainers must accept more black boxes.
In some AI systems – especially those using personal data or those where proprietary information is at stake – the demand for explainability may interact with concerns about privacy. In areas such as healthcare and finance, for example, an AI system might be analyzing sensitive personal data to make a decision or recommendation. In determining the type of explainability that is desirable in these cases, organizations using AI will need to take into account the extent to which different forms of transparency might result in the release of sensitive insights about individuals or expose vulnerable groups to harm.
In the area of maintenance, when the AI recommends a maintenance decision, decision makers need to understand the underlying reason. Maintenance analytics developers need to understand what fault features in the input data are guiding the algorithm before accepting auto-generated diagnosis reports, and the maintenance engineer needs to understand which abnormal phenomena are captured by the inference algorithm before following the repair recommendations.
One of the proposed benefits of increasing the explainability of AI systems is increased trust in the system. If maintainers understand what led to an AI-generated decision or recommendation, they will be more confident in its outputs. But the link between explanations and trust is complex. If a system produces convincing but misleading explanations, users might develop a false sense of confidence or understanding. They might have too much confidence in the effectiveness or safety of systems, without such confidence being justified. Explanations might help increase trust in the short term, but they do not necessarily help create systems that generate trustworthy outputs or ensure that those deploying the system make trustworthy claims about its capabilities.
Authors: Uday Kumar, Diego Galar and Ramin Karim, Luleå University of Technology
References:
Galar, Diego, Pasquale Daponte, and Uday Kumar. Handbook of Industry 4.0 and SMART Systems. CRC Press, 2019.
Galar, Diego. Artificial intelligence tools: decision support systems in condition monitoring and diagnosis. Crc Press, 2015.
Galar, Diego, Uday Kumar, and Dammika Seneviratne. Robots, Drones, UAVs and UGVs for Operation and Maintenance. CRC Press, 2020.