Combining Machine and Human Intelligence to Unlock Hidden Value in Existing Process Data
Industrial companies are under pressure to drive value with advanced analytics and machine learning. The promise of these technologies is compelling, but as always, the devil lies in the details – which in the heavy industry context often include the availability and quality of suitable data.
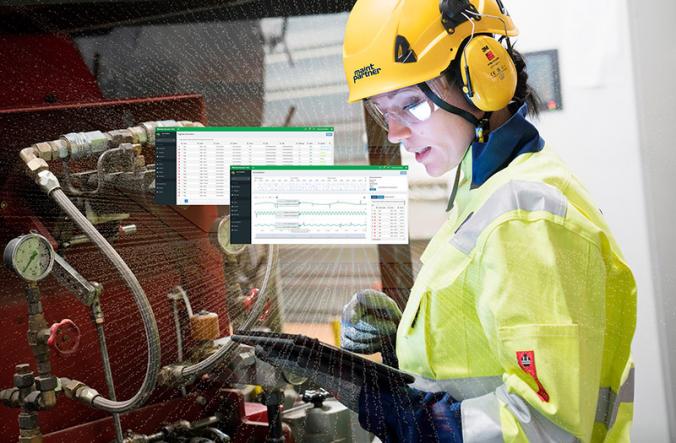
Companies wonder if their historical CMMS data is accurate enough, new sensors should be installed, or an IoT platform to visualize new measurements needs be deployed. These are frequent and relevant questions when considering how to apply AI and analytics in industrial O&M. But, at the same time the opportunity to work with high-quality, accurate and automatically recorded data already available might be overlooked. We are talking about the process data in the DCS or other information systems.
According to Maintpartner’s digital solutions experts, there is plenty of untapped potential in that data. With strong analytics capabilities and the right approach involving the process specialists, early signs of emerging equipment failures can be identified well before they escalate – or suboptimal operation of the can be process spotted. Such online analytics can work as an insurance against surprise downtime, help shift from unplanned to planned works and eliminate all sources of functional or operational underperformance 24/7/365.
Familiar data – advanced analytics
Monitoring of signals against static upper and lower bounds is easy and widely used. But what about combinations of several signals; what are normal values for each in different operating conditions and situations? What about the time dimension; how long does it take for actuators to react to controls or to complete a motion from one extreme position to another? This is where advanced analytics is required to monitor and draw new insights from the existing data.
Such complex connections and time-series patterns, and abnormalities in these patterns in particular, are often the early signs of emerging problems that today seem to occur without any advance warning. The warning signs would have been there, hidden in the data, and advanced analytics can make them visible and actionable early enough to have significant business impact. This ability to identify potential issues early enough to take a new course of action is what differentiates really advanced analytics, thus making them relevant to business and top management.
Tacit knowledge capture
Computer algorithms are good at crunching numbers. To some extent they can also “learn” almost independently by adjusting their parameters based on a well-defined reward function or annotated sample data. However, they cannot think independently and need human intelligence to get started and to become better.
A data-driven, human-guided modeling approach takes a broad scope, e.g., thousands of signals from a whole plant, and does a rough clustering of the tag list based on the main sub-processes. A light interview about the specific process characteristics and typical challenges opens a fast lane to well-structured models right from the beginning. Unsupervised machine learning digests a training data period of all process measurements and learns the correlations and dynamics between the signals in different operational situations. Now the system is ready for online monitoring of live data and it starts to raise warnings about abnormal behavior relative to what it has “seen” in the training data. Human experts who know their process then rate these warnings to be either true positive (relevant) or false positive (accepted behavior), and this rating information is fed back to model retraining. Thus, the model learns from human feedback on real-time situations and converges towards giving only correct and all correct warnings.
When such feedback is collected from highly knowledgeable experts, either as the warning ratings or interviewed feed to other analytical models, the tacit knowledge is captured into the AI. The AI is able to share these insights across the whole operator crew, which may be highly relevant considering, e.g., retirement of the legacy experts or unifying operator shift performance.
Learning curve to drive value
Industrial AI is already moving beyond proof-of-concepts to being delivered as continuous operational services in selected applications. The focus should be on how the new applications drive value creation rather than testing how specific techniques and technologies work.
In industrial O&M, where human decisions and actions are still in the loop for the foreseeable future, real value is not created by the new application alone. It is at least as much about how the organization uses the new (analytical) support available, how it flows into the daily practice of the O&M experts, planners and operators. Take anomaly detection as an example: who has the authority and responsibility to act based on what the machine suggests, how much evidence is required to be sure to act upon a warning or move from time-based to condition-based maintenance, how the systems are used in practice, how reporting is done, and so forth.
It takes commitment from the organization and requires solid change management to really make the new applications and new ways of working with the data an everyday practice. And only then one creates sustainable value, instead of just running a series of isolated exercises. Despite all the talk about overwhelming transformation, the AI is not a magic wand – it takes some patience to drive it to the finish line, but the journey is a valuable learning path itself and the end result is better adopted when the user team has been involved in the validation journey.
Luckily, by tapping to the existing data, getting started takes very little time and upfront investment. Once you learn what your existing data can tell you, you can decide to invest into acquiring additional data. And then you already know how to use it valuably.
Maintpartner at the forefront
One of the players in the field is the Finland-based Maintpartner. The company has delivered its MP INtelligence® advanced analytics service for several years, productizing cutting-edge algorithmic development that dates back by more than a decade.
We believe in learning by doing in real industrial environments. We have already gained valuable knowledge on how to deploy advanced anomaly detection in energy, steel, pharmaceutical, aviation and pulp & paper industries. The value creation is at the core of our value proposition and our customers are realizing significant value through our actionable insights. As an example, one of our customers estimated 1.7-2.5 M€ of avoided unavailability costs in 12 months.
MP INtelligence® is a service concept powered by AI and machine learning. It has been developed with the customers in solving real-world problems. Maintpartner’s experienced team delivers a streamlined implementation process from data connections to operational online models, with constant backend support from the Control Tower. MP Intelligence’s data-driven, human-guided model has been proven to work and is fast to implement. Online model validation can begin in just a few weeks from the kick-off via the web UI, with a scope that covers even the whole plant.