How ultrasonic sensors and artificial intelligence improve condition monitoring
In an industrial setting, assets are everything. A breakdown can cause hours of downtime, and thus hours of lost productivity and lower financial gains. Preventative maintenance is one way facility managers counteract system entropy, but it is not perfect strategy. In some cases, a preventive approach to maintenance can actually lower the overall effectiveness of an asset. In the case of a valve for example, constant tightening can cause premature wear and tear.
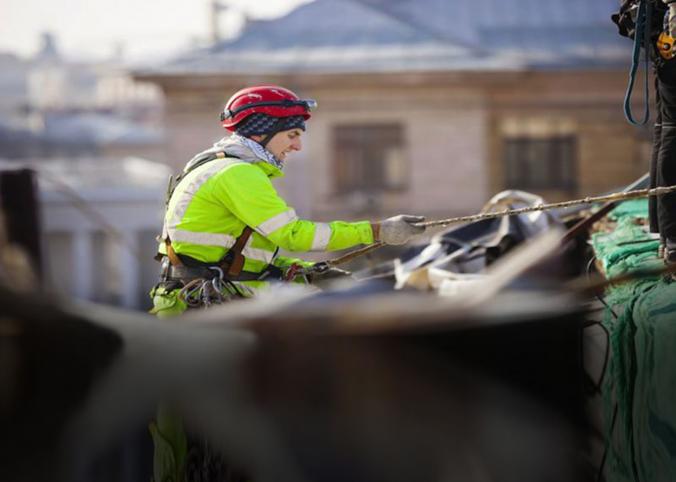
Predictive maintenance was the next logical step forward. Rather than scheduling maintenance, this method anticipates failure and only takes action when it is most necessary. This too has its downfalls, such as when an asset is so complex that it becomes impossible to predict every impending failure.
Now, facility managers can use sensors, machine learning and artificial intelligence to predict failure states with a much higher degree of accuracy. Implementing a smart monitoring system takes time and considerable effort – but when dealing with multi-million-dollar assets, the value becomes obvious.
Complex assets are resistant to traditional condition monitoring
When a pharmaceutical company reached out to Blair Fraser's team at Lakeside Process Controls, they wanted to improve the uptime of a critical industrial asset, an industrial autoclave.
- It was a simple request from our client to improve the reliability and uptime of this industrial asset. The client identified, through risk assessment, what could have the biggest impact on production, Fraser noted.
However, that goal turned out to be more complex than anticipated. The autoclave featured hundreds of critical components.
- We started out by doing failure mode and effect analysis, which is a way of looking at an asset and breaking it down into its components and then determining how that asset could fail. In doing that, we identified about 260 ways that an asset could fail. About 150 of those could be mitigated through condition monitoring, Fraser said.
- It is impossible for humans to monitor complex assets.
Through assessments, the team identified three components within the autoclave that would have the biggest impact on future productivity: steam traps, diaphragm valves and the vacuum pump. However, even narrowing the problem down to a single component proved to be a problem, considering the asset could operate in 13 different ways.
Traditional condition monitoring works well for continuous assets that consistently output a regular stream of data. Complex assets with several dozen variables on the other hand, cannot be monitored in the same way. Industrial Internet of Things (IIOT) sensors have made some progress toward a more granular approach to asset condition monitoring, but they still require human intervention. And when an asset outputs variable data faster than a human can comprehend, it is all but impossible to produce value from that data.
In the case of the industrial autoclave, traditional condition monitoring was not reliable enough to accurately predict asset failure. There were simply too many variables.
Controlled scenarios versus real world applications
The first step toward creating an effective condition monitoring strategy is to determine a baseline of optimal functionality. In other words, before you can determine if an asset is about to fail, you need to know what it looks like when it is in perfect condition.
For example, the team at Lakeside Process Controls brought in a diaphragm valve to test at their own lab. They attached an Ultra-Trak™ 750 to monitor ultrasonic amplitude. With the sensor in place, they benchmarked the valve in proper working order, then induced a failure to see how the data output changed. In this way, the team was able to see how the ultrasonic readings changed when the valve was in various states of failure.
However, in a real-world setting, the team found it was impossible to reproduce the results they produced in the lab. Too many ambient factors affected the diaphragm valve to be able to take an accurate reading while working.
• Using manual root-based condition monitoring technology, we found that it was nearly impossible to get good, repeatable data. In order for condition monitoring technology to work, we need to have repeatable data, said Fraser.
When the team gave their records to a data scientist, they found that each component in the autoclave had a factorial of 32. In other words, at any given second, each of the roughly 50 pieces could display 32 different readings, in a wide decibel range. It might take a human months to go through a single minute of data, and even more time to develop failure alert rules based on that information.
In an industrial setting, assets are everything.
To monitor conditions in such a complex asset, Fraser's team needed a better way to track variables in real time. They needed a solution that could do what a human could not. That is when they engaged Quartic.ai, an IIoT and Industrial AI company.
How machine learning and artificial intelligence improve first-pass analysis
Imagine that you could put your best engineer in a room with a single component of your most valuable asset and have them constantly monitor and assess its condition – forever. With the Ultra-Trak™ 750 and a trained AI, that becomes a reality.
Fraser's team recorded months of data from 'golden batches' – that is, perfect runs of an autoclave cycle. Quartic.ai used data from several batches of different recipes and during the idle state, to train machine-learning algorithms that capture the "baseline" patterns for each ultrasound signal under different operating states. They gave the data to an artificial intelligence company that trained a machine-learning algorithm to understand when monitored components are operating perfectly. Then, the newly formed AI monitored real-time data from the asset. Every second, the AI compares data from 50 components to its baseline, then gives an anomaly score between zero and 100. A score of zero means the real time data exactly matches the baseline. A score of 100 means the data is the exact opposite of the baseline. A prolonged high score indicates impending failure.
The AI did what no human could do. In a real world scenario, the algorithm correctly identified asset failure two hours before the rule-based auto-shutdown activated.
In the future ultrasonic sensors and machine learning algorithms will be able to monitor ever more complex assets, potentially saving thousands of hours in downtime and fully optimizing maintenance routines. The applications of this smart solution are endless, from industrial plants to commercial assets.
In the case of the pharmaceutical industry, this technology will mean fewer lost batches and potentially millions in saved dollars.